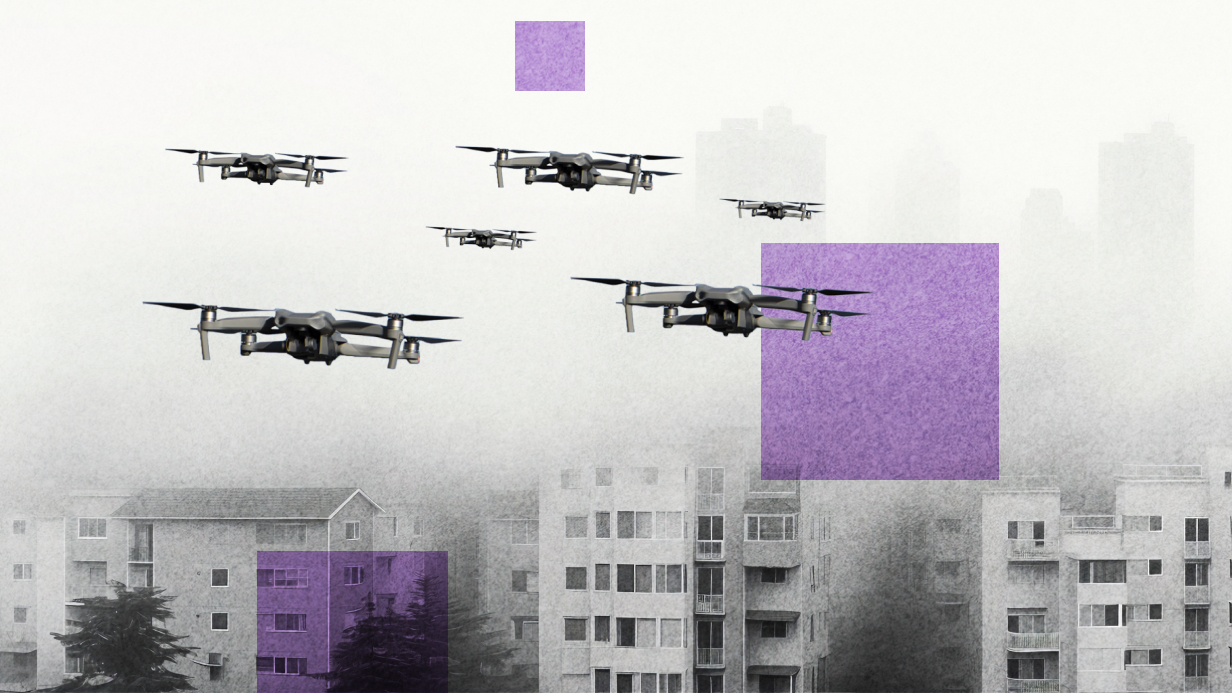
Behind the facade of Putin’s discussions with technocrats about the threats posed by Western generative chatbots lies the full-scale militarization of Russia’s artificial intelligence sector. T-invariant examines how Russian forces are already using AI to guide kamikaze drones via optical navigation (immune to enemy electronic warfare), refine combat tactics with drone swarms, overhaul military logistics, and repurpose cryptography—now geared more toward cyberattacks than data protection. While Russia lags in the civilian AI race—losing ground in both exascale supercomputing and large language models (LLMs)—its “sovereign AI” prioritizes military applications where massive computing power is less critical. In this shadow war, Russia has ample intellectual and computational resources.
The rhetoric about AI’s role in warfare has become a recurring theme from both sides of the new Cold War. Just recently, Elon Musk warned that “if a war breaks out between major powers, it will be a war of drones and artificial intelligence,” expressing concerns this could lead to “the creation of Terminator”-like scenarios. He urged the U.S. Military Academy at West Point to help prevent such outcomes while calling for increased drone production in America. Similar discussions dominate Russia’s political discourse. High-ranking officials, for instance, have convened government meetings to examine “how artificial intelligence can enhance combat effectiveness in active war zones.”
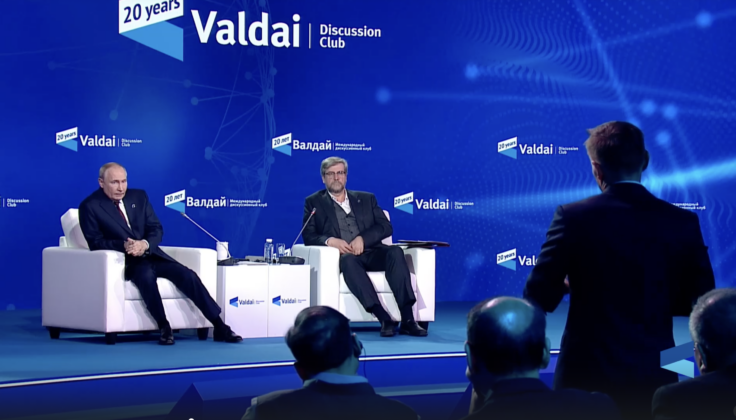
Sovereign Artificial Intelligence
Russia is advancing a technology it calls “sovereign artificial intelligence”—a term that, while it may sound like propaganda, is rooted in substantive strategic goals (which we will explore further). On one hand, the war hinders AI development; on the other, it accelerates it, particularly in military AI models, where “no expense is spared.” Given that our focus here is on military AI applications, little information is publicly available—a reality not unique to Russia but shared by the U.S., China, Ukraine, and others.
The term “sovereign AI” was coined by Russian President Vladimir Putin, gaining traction after his remarks at the Valdai Club meeting in Sochi on November 7, 2024. During the same event, Ruslan Yunusov, co-founder of the Russian Quantum Center, raised alarms about generative chatbots promoting “left-liberal agendas” and negatively influencing Russian youth.
“In recent years, warned Yunusov, we’ve seen AI increasingly trained on synthetic rather than real-world data, which risks radicalizing these models. But far more dangerous is AI’s subtle role in shaping young minds, implanting ideologies that often originate abroad—particularly across the ocean. While tighter regulation is necessary, outright bans won’t work. Instead, we must nurture domestic AI technologies. Fortunately, Russia has made significant progress here. In fact, we are one of just three nations with a full stack of IT capabilities—the true foundation of sovereignty.”
Whether Russia truly possesses a “full IT stack” is debatable. Without domestic high-end processor production, claims of technological independence are dubious. Yunusov likely knows this. In reality, only one country has a complete IT stack, and even it relies on international cooperation.
Putin later took the floor, acknowledging AI’s dual nature—like nuclear energy, it carries risks but also benefits. He stated, “Banning it, I think, is impossible. But one way or another, it will find its way—especially under competitive conditions. Competition is intensifying. I’m not talking about armed confrontation now, but broadly in the economy, competition is growing. So in the context of this competitive struggle, the development of artificial intelligence is inevitable. And here, of course, we can be among the leaders, given the certain advantages we possess. As for sovereignty—it’s a critical component. Yes, these platforms are mostly developed abroad, and they shape worldviews—absolutely correct. Here, we must recognize this and develop our own sovereign artificial intelligence. Of course, we should use everything available, but we must also advance our own initiatives in this field. Our companies—Sber, Yandex—are actively working on this, and overall, they’re doing quite successfully. We will undoubtedly pursue all of this; there’s no question about it, especially in areas where it’s already self-replicating—that’s extremely interesting and promising.”
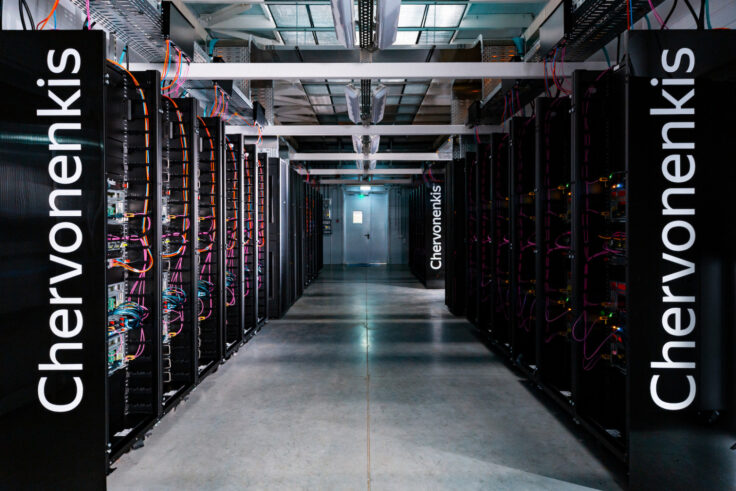
The meaning of “self-replicating” remains unclear—possibly referencing synthetic data training, which Yunusov warned could lead to model collapse. But Putin’s key takeaway was: “Competition is intensifying. I’m not speaking of armed confrontation, but economic rivalry makes AI development inevitable.” In this race, failing to develop weapons your adversaries are advancing means defeat. And while Putin avoided mentioning “armed confrontation” at Valdai, Russia’s military AI ambitions are unmistakable. But how accurate is the claim that “we can certainly be among the leaders here, given the certain advantages we possess”—and what exactly are these advantages?
Most likely, they refer to AI models developed over years by Yandex, Sber, and possibly other companies and organizations that prefer to operate outside the public eye.
Top-Down Constraints
Developing AI models—generative, navigational, or otherwise—requires substantial funding and cutting-edge technology.
How Were These Problems Solved Before the Full-Scale Invasion of Ukraine and the Imposition of Sanctions? Russia’s tech giants like Yandex and Sber had a straightforward approach – purchasing foreign hardware. They bought everything needed for supercomputers off the shelf—Nvidia or Intel GPUs, Infiniband/Ethernet interconnects, storage drives, cooling systems, and other critical components (which, despite seeming like minor details, were actually precision-engineered systems). These parts were imported, assembled, and configured into fully operational machines.
Then choosing the software stack. Yandex developed its own architecture for AI training. Sber, on the other hand, often relied on American frameworks (e.g., TensorFlow, PyTorch) and even used Microsoft Azure for additional computing power. For example, Sber’s “Kristofari” supercomputer had a gateway connecting it to Azure for extra capacity.
They had no shortage of resources. If a company had the budget (say, for 10,000+ Nvidia GPUs), there were no obstacles—vendors delivered, installed, and fine-tuned everything. Need more power? Rent cloud capacity from Google or Microsoft.
Russia never seriously pursued developing its own semiconductor base or building competitive GPUs. The country’s lag in this field is enormous, and closing the gap today is virtually impossible—despite occasional rhetoric about the need for local chip fabs. Moore’s Law dictates that each processor generation doubles not only computing power but also fabrication costs. Building a competitive fab requires tens of billions of dollars—unfeasible without global market access. Even a large domestic market like Russia’s can’t justify the expense. To break even, a Russian fab would need to compete globally, but the semiconductor industry is already dominated by entrenched players (TSMC, Intel, Samsung). High Risk, No Guaranteed Reward.
Moreover, today there are no cutting-edge processors manufactured by a single country alone. For instance, the Dutch company ASML produces photolithography machines, but the actual processors are made by TSMC in Taiwan. These are all multibillion-dollar technologies. That’s precisely why no one even considered developing Russia’s own processor production in the first place.
The initial reaction to the sanctions against Russia after February 24 was one of shock. It turned out that nearly all semiconductor supply chains had been severed, and even relatively modern domestic processors like the “Baikal” were still being produced in Taiwan. However, the shock subsided, and a “gray” import market gradually emerged. Simple processors are produced by the billions worldwide, making it virtually impossible to halt their flow into Russia. Small, fly-by-night wholesale firms set up chip supply channels—not just for the most basic models but even advanced ones like the Nvidia H100. Hundreds of thousands of these chips are manufactured, and tracking every single batch is no easy task. (The situation is even simpler with processors like Intel Xeon: there are millions of them, but they’re general-purpose chips not optimized for AI.) This is exactly how the “MGU-270” supercomputer was assembled. So far, there’s no sign of Russia making any progress toward producing its own chips of this caliber. China doesn’t know how to make them either—at least not at an industrial scale—and even if it learns, there’s no guarantee it will supply them to Russia.
However, it’s important to note that tracking chips—such as GPUs—as they disperse across the global market is extremely difficult precisely because their numbers grow so large. And their numbers grow when the technology not only matures and scales but also becomes obsolete, transitioning from today’s cutting-edge to yesterday’s top-tier. So even if gray imports function well, they deal in older models. By relying on this form of procurement, Russia inherently accepts a 2–3-year lag. It’s workable, but always a couple of steps behind. If the demand isn’t for 10,000 processors but, say, 200,000 H100s—or the very latest H200s, like those in Elon Musk’s “Colossus” supercomputer—problems arise again: gray-market schemes are no substitute for direct supply. They’re unreliable and limited in volume. When quantities spike, those enforcing sanctions take notice and shut down the pipeline. Another workaround may emerge eventually, but that’s a problem for later.
With software, on the other hand, things are relatively stable. For AI development, there are two critical libraries—PyTorch and TensorFlow. They are tightly integrated with processor architectures, including Nvidia’s. However, the libraries themselves are open-source, and Nvidia’s drivers and specialized libraries (CUDA) are freely available. Issues may arise with cloud access, but they are solvable.
In general, if you’re an average developer, this isn’t your problem. Yandex Cloud or Cloud.ru are ready to maintain and update libraries, as well as ensure compatibility with the latest Nvidia chips—assuming, of course, you can get your hands on them.
But overall, this means that lag is built into the system. And for the most advanced AI models, this is critical. A simple example: take ChatGPT-3.5 and compare its performance with ChatGPT-4o—even just the free version. You’ll immediately notice the difference. And between them lies a two-year gap.
However, Russia may still develop AI models—and secure funding, processors, and software. No, Russia isn’t competing in the “AGI race” (that is, the pursuit of the most advanced, near-human-like “general” AI), nor can it under current conditions. (Here, all the talk about “who even needs AGI” is pure Aesop—the fox declaring the grapes sour.) But AI is a vast field, and in some areas, the “sovereign AI” Russia is building could end up on the cutting edge. After all, it’s being designed precisely for the “frontlines.” The requirements here are different, and so are the chips needed—not Nvidia’s H100, but Nvidia Jetson or even more advanced solutions with programmable logic (like AMD Xilinx processors).
The Exaflop Race
Russia is waging a war—an extremely costly one. According to various estimates, over three years, Russia has funneled around $300 billion into the war effort across different fronts. Moscow does not disclose these expenses, but the Pentagon estimates $211 billion spent in just the first two years (as of February 2024). It’s unlikely that spending decreased in the following year, so an annual estimate of $100 billion seems realistic. Is that a lot? By any measure, it’s an astronomical sum—but not in today’s AI race.
The publicly announced investment plan for Project Stargate—a private initiative led by SoftBank and OpenAI—stands at $500 billion over five years. Its unstated goal? The development of AGI (Artificial General Intelligence)—a concept nobody truly understands, yet one that’s already being built. And this is just one project among many. There’s an entire ecosystem of companies with market caps nearing $100 billion and tens of billions in funding, all racing to advance AI models. (Google, x.AI—Musk’s company building Colossus—and other tech giants aren’t even part of Stargate.) In total, global AI investments are approaching $1 trillion. Last year, Sam Altman estimated that $5-7 trillion would be needed just for AI chip production. At this scale, even Microsoft’s Three Mile Island nuclear plant reboot—aimed at powering data centers—looks like a speck of dust on a boot. Russia simply doesn’t have that kind of money, and under wartime conditions, there’s nowhere to get it. China’s position is also uncertain—while its semiconductor situation is far better than Russia’s, it’s still nowhere near that of U.S. firms, especially under sanctions.
AI is becoming prohibitively expensive. “Nature” recently highlighted OpenAI’s o3 model, noting: “o3 is already resource-intensive: to solve a single task in the ARC-AGI benchmark, it took an average of 14 minutes—likely costing thousands of dollars per attempt.” This is a far cry from seconds-long responses or even the $20–200/month subscriptions for models like Deep Researcher.
Why Are American Companies Investing Such Vast Sums in AI? Trump Believes It’s a Matter of National Security (He Personally Endorsed Stargate). The issue at hand is not the risks associated with AI development and deployment, which dominated discussions over the past year—when the need to regulate AI advancements was repeatedly debated in Congress, the Biden administration, and the EU. Rather, it is about America’s security, which, in the view of Trump’s incoming administration, is threatened by the progress of military AI, primarily in China.
Once Again, War Seems to Be the Driving Force Behind Scientific and Technological Progress. And AI Is the Priority. However, while war remains a hypothetical scenario for the United States, for Russia, it is a daily reality. Russia cannot afford to engage in a global race with long-term and uncertain outcomes, such as the development of AGI (Artificial General Intelligence). What Russia needs are concrete, actionable solutions—today.
As “T-invariant” reported on the launch of the “MSU-270” supercomputer: “The flagship academic supercomputer will specialize in artificial intelligence, with the dedicated AI Institute at Moscow State University headed by Katerina Tikhonova—Putin’s daughter. The high-ranking academic leader acknowledges that Russia is currently ‘following trends rather than setting them’ in this ‘exaflop race.’ However, Tikhonova argues that the country has a ‘more pragmatic approach to AI development,’ one that is ‘shaped by the tasks and challenges facing Russia’—namely, ‘applications in UAVs (unmanned aerial vehicles) and the oil and gas sector.’”
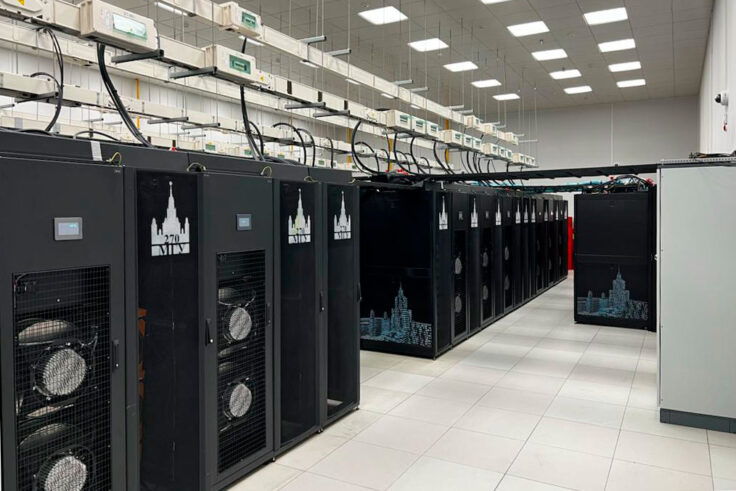
Tikhonova has a realistic view of AI development in Russia. What has transformed modern warfare? The answer is well known—drones. These include unmanned surface and underwater vehicles, but the most significant are UAVs (unmanned aerial vehicles), capable of carrying lethal payloads and operating either under remote human control or autonomously, individually or in swarms.
In the 19th century, as the density and range of precision gunfire increased, armies abandoned column-based assaults. In the 21st century, with the advent of drones, militaries no longer concentrate large reserves at the focal point of an offensive—a classic tactic of war—but instead rely on rapidly deployable mobile strike units. However, for a drone to operate autonomously deep behind enemy lines, it must be equipped with AI.
Beyond combat applications, drones also play a key role in solving logistical challenges, and their rise has reshaped traditional cryptography tasks with the integration of AI.
One final point: had Russia not been at war, it might have had the resources to compete in the “exaflop race.” But today, the country has different priorities.
The End of the “Guerrilla” Era in Drone Development
First, we must clarify that the specific tactical and technical characteristics of UAVs (commonly referred to as “drones” or “unmanned vehicles”) remain largely undisclosed or are known only in approximate terms. Our focus here is not on drones in general, but rather on one narrow domain: the application of AI models in military drones.
Of course, a drone is more than just an AI model. In fact, AI integration currently represents the future—a future that is being actively tested and is likely very near, but still the future. Today, most frontline drones do not yet utilize AI models. But there is no doubt that they soon will. Scalability of military technology hinges critically on cost. An advanced drone chip costs thousands of dollars and is difficult to procure through “gray imports” – let alone deploy and scale in production. Establishing stable manufacturing becomes impossible when components are available today, gone tomorrow, and then reappear the day after as technically identical yet different batches. Sanctions play a substantial restraining role here, an impact that shouldn’t be underestimated. While for Ukraine, acquiring Nvidia Jetson processors – specifically designed for mobile (including autonomous) robotics – is merely a financial challenge, Russia faces far greater constraints.
But first, let’s briefly examine the current military application of drones. Here, we’ll reference a December 2024 report published by the “Institute for the Study of War (ISW)”.
Both Ukraine and Russia are establishing new military branches dedicated to unmanned systems. Ukraine has formed the “Unmanned Systems Forces (USF)”, while Putin has announced a similar initiative in Russia. Currently, in both countries, drone warfare remains heavily reliant on private initiatives. The Wagner Group pioneered large-scale drone use during the Battle of Bakhmut, with volunteers and private funds handling both assembly and operator training. Often, drones are deployed in combat zones despite objections from field commanders.
However, Russia’s Ministry of Defense is now consolidating these efforts into a dedicated branch (a distinct military service). On one hand, this restructuring is fraught with challenges—disrupting established production and deployment mechanisms mid-war. On the other, Russian experts estimate that within 6–12 months, this reorganization could significantly enhance drone capabilities and expand their combat roles, including the development of advanced, expensive UAVs that cannot be improvised in makeshift workshops or operated without specialized training.
Putin’s statements, Tikhonova’s remarks, and Defense Minister Belousov’s August 2024 creation of the “Rubicon Center for Advanced Drone Technologies” all signal an impending transformation in Russia’s combat drone forces. The “guerrilla” era of ad hoc drone warfare is ending. Russia appears willing to accept short-term tactical disruptions, including reduced battlefield cohesion, to secure a strategic advantage in the near future.
Russia’s military advances in UAV technology are now being propelled by the country’s top universities and leading innovation hubs. The Moscow Institute of Physics and Technology (MFTI) and ITMO University have emerged as pioneers, establishing think tanks specializing in “drone swarm” technology – a cutting-edge direction in military drone development. Read more in T-invariant’s report: “”Aerokitties” Fly in Swarms”
AI Models in Warfare
A drone is an unmanned system designed to perform multiple tasks. First and foremost, it must be able to fly—preferably, all drones deployed to the frontlines should remain airborne (currently, up to a third of drones in large shipments may fail to launch or crash immediately, a key reason behind the Russian Defense Ministry’s strategic shift).
To take flight, a drone requires either an electric motor or an internal combustion engine (ICE). For instance, Russia’s “Geran-2” (an analog of Iran’s “Shahed”) uses a two-stroke ICE. Both propulsion systems and batteries are constantly improving: engines are becoming more efficient, while batteries deliver longer endurance.
Once airborne, the drone must reach its target and complete its mission despite enemy countermeasures. It can operate either autonomously or under an operator’s control.
Navigation is the primary task where AI models prove decisive today. Commercial drones typically rely on satellite navigation (e.g., GPS) to chart and follow predetermined routes. Military drones, however, cannot depend solely on these systems due to electronic warfare (EW) threats like:
– Jamming: Disrupting radio frequencies used for drone-operator communication. Simple drones using a single frequency are most vulnerable.
– Spoofing: Hijacking or falsifying GPS signals to misdirect the drone.
Advanced drones employ frequency-hopping to evade jamming, though blanket interference across entire bandwidths risks disrupting friendly systems. For basic drones, jamming alone often suffices to neutralize them. Single-frequency drones can be easily disabled by targeted jamming. Frequency-hopping models require multi-band interference, complicating EW efforts. GPS spoofing forces drones to rely on backup navigation (e.g., inertial systems or AI-driven visual terrain recognition). The vulnerability of GPS-dependent systems has accelerated AI adoption for alternative navigation—such as optical/terrain-based algorithms—making drones less reliant on satellite signals. This shift is reshaping both drone design and electronic warfare tactics on the modern battlefield.
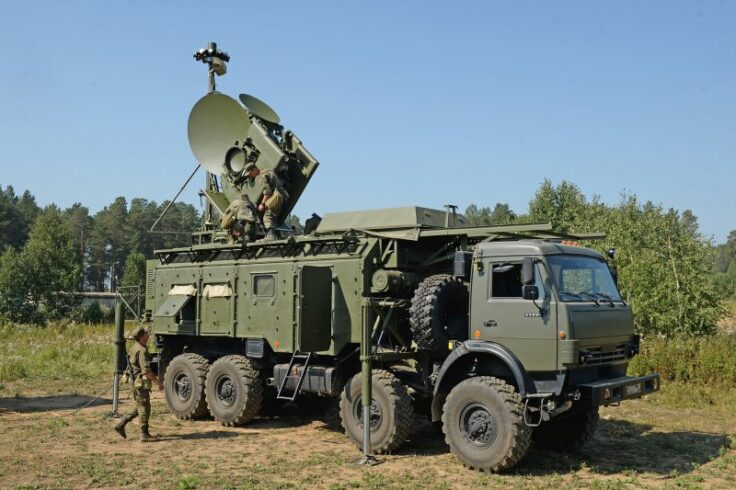
While jamming disrupts signals, spoofing is far more sophisticated—and dangerous. By forging GPS signals, attackers can hijack a drone’s navigation, causing it to lose its trajectory (drifting off course), to reverse direction (flying “home” while actually heading toward enemy territory) or to miscalculate strikes (misidentifying targets due to falsified coordinates).
The “Geran-2” drone employs a two-tiered approach: GPS Navigation (outside EW zones) for standard satellite guidance for precision in uncontested airspace, and Inertial Navigation System (INS) (inside EW zones). The system records data from gyroscopes, which track all rotational movements of the device, and an accelerometer, which measures linear acceleration. Based on these inputs, the processor calculates changes in position and plots the trajectory.
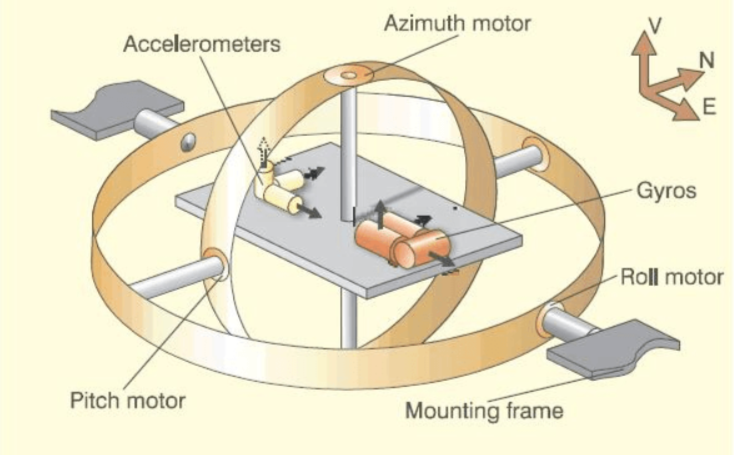
The primary limitation of inertial navigation systems (INS) is that the drone’s actual position gradually diverges from its calculated position over time. Periodic position calibration is required. However, since electronic warfare (EW) deployment zones are typically small, basic INS systems are often sufficient. This is how the “Geran-2” operates, as do most military drones today. Yet the system is far from perfect—due to both the inherent instability of INS and its reliance on GPS.
For a drone to engage a target, it must first detect it. The simplest scenario involves static targets: the drone attacks not the object itself, but a pre-programmed point on the map. If navigation calculations are correct and GPS functions properly, the target will likely be hit with reasonable accuracy. To improve strike precision, the “Geran-2” employs a thermal imager (infrared radiation detection) and an optical camera. Autonomous target recognition requires some form of computer vision—today, this can be achieved using AI models. The “Geran-2” likely utilizes a similar approach, much like Russia’s “Lancet” loitering munition.
Is it possible to operate entirely without GPS? Yes. Several technologies have been developed for this purpose, including magnetic navigation, celestial navigation, and optical navigation—each with its own advantages and limitations. An ideal navigation system would integrate multiple methods, dynamically switching between them as environmental conditions change.
Magnetic navigation relies on a detailed geomagnetic map of the terrain. Earth’s magnetic field varies in both intensity and direction from one location to another. By charting these variations, a drone equipped with a magnetic sensor can detect local fluctuations. An AI model then cross-references real-time sensor data with the preloaded magnetic map to determine the drone’s position.
So far, no mass-produced drones are known to use this system, but prototypes are under development, including in the U.S. A major limitation is the lack of high-resolution geomagnetic maps for all regions. Some systems supplement magnetic sensors with gravimetric ones to enhance accuracy.
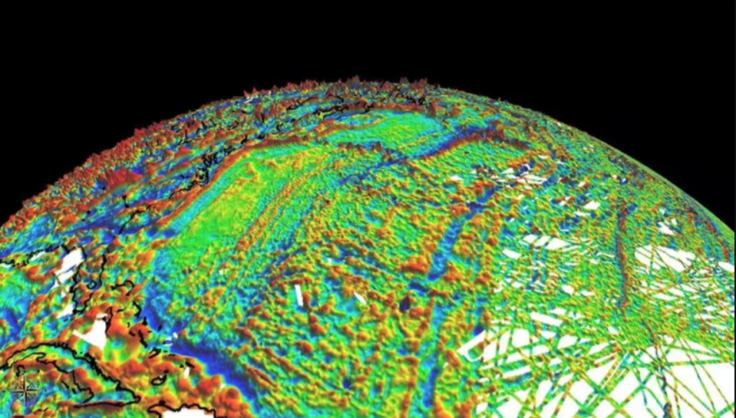
Russia’s “Burevestnik” cruise missile likely employs celestial navigation. The principle is straightforward: the missile orients itself by referencing stars. However, this approach has an inherent limitation—stellar visibility is not guaranteed in all conditions (e.g., during daylight or under heavy cloud cover).
Optical Navigation is an AI-powered alternative. This method leverages artificial intelligence for fully autonomous operation. The drone is preloaded with a high-resolution optical map (typically satellite imagery) and continuously matches real-time visuals with this reference data. CNN (Convolutional Neural Network) identifies terrain features and objects, RNN (Recurrent Neural Network) handles route planning and trajectory optimization. Supplementary AI models perform motion prediction, dynamic path adjustment, and other navigational tasks (all currently existing technologies).
The system is fully autonomous and requires neither GPS nor communication with an operator—it makes all decisions independently, including target acquisition and strike timing. This drone is equally effective against moving targets, which it identifies using thermal imaging (e.g., at night) or visual recognition. It can be deployed both near the front lines (as it is immune to electronic warfare systems) and for deep strikes into enemy territory. The entire system is resilient to environmental changes: even if a forest has burned down or a bridge has been destroyed, it can typically determine its subsequent flight path.
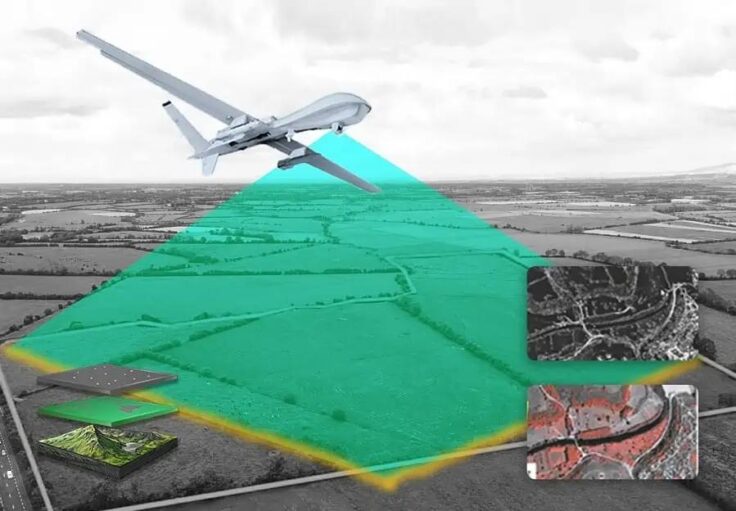
Neural networks are trained on high-performance machines (the “MGU-270” is fully suitable), but they do not require cloud systems or supercomputers for operation. However, they do need fairly powerful chips. These could be Nvidia Jetson, but even better are programmable logic chips, such as the AMD Xilinx Zynq UltraScale+, which enable real-time neural network processing. Such systems cannot be built in a hobbyist workshop. These drones are expensive but could likely provide a military edge. Nvidia Jetson and AMD Xilinx Zynq UltraScale+ are under sanctions, but gray-market imports remain a viable workaround.
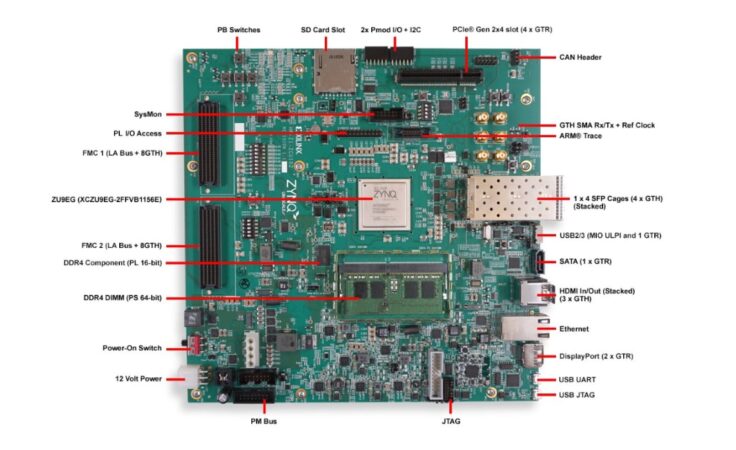
“Geran-2” is a kamikaze drone. It is a loitering munition designed to deliver its payload to the target. Using expensive, sophisticated drones for one-way missions is not cost-effective. However, requiring a drone to return to base complicates its operation and limits its penetration depth. Nevertheless, it appears that such reusable drones will be the focus of future development.
So far, we have only discussed individual drones carrying out combat missions autonomously. But today, one of the most promising areas in military drone development is drone swarms—coordinated operations involving large numbers of unmanned systems, both in attack and defense.
Most people have likely seen drone light shows (if not in person, then on YouTube), where thousands of drones form intricate 3D shapes in the sky. These displays are visually stunning but rely on a centralized control system that directs each drone’s movement and ensures synchronization. For example, “Geoscan”’s drone shows operate this way. Occasionally, drones malfunction and rain down on spectators—hardly a safe scenario.
However, military drone swarms operate under much harsher conditions. While the main challenge in a light show is collision avoidance, in combat, drones must maintain cohesion despite EW and active enemy countermeasures.
The simplest method mirrors drone shows: a centralized control hub where an operator manages the entire swarm rather than individual drones. The drones coordinate their positioning and follow commands from the center. However, this approach faces the same EW vulnerabilities (jamming and spoofing) as single-drone operations.
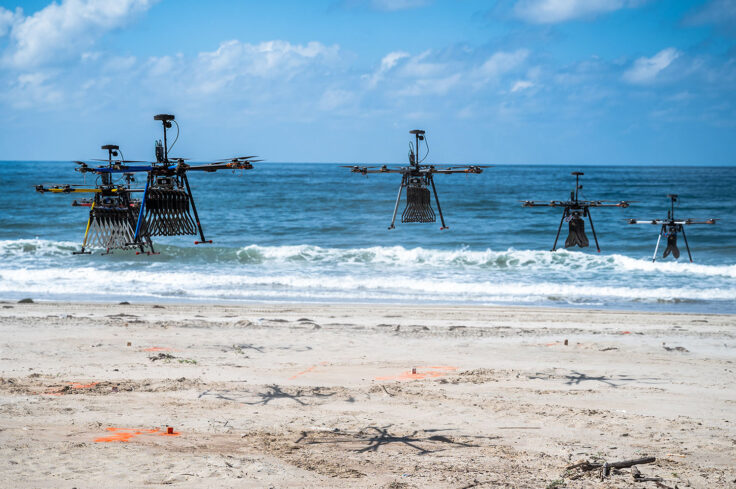
However, other solutions exist—particularly those leveraging AI models for fully autonomous swarm control. One method involves a centralized server system, where one or several “leader” drones act as command nodes, directing the rest of the swarm. Yet this approach remains vulnerable to EW countermeasures. A more resilient alternative takes inspiration from nature: drones could coordinate mission execution by observing nearby units, much like birds in a flock. While no deployed systems currently use this method, digital prototypes are under active development.
Stopping such a swarm is extremely difficult, if not impossible—unless intercepted by another swarm. To date, no air battles between opposing drone swarms have been documented. However, managing these swarms demands highly advanced AI models capable of real-time decision-making. Examples include Palantir AI, a battlefield management system, while Rostec is reportedly attempting to develop a similar capability.
The Military Transport Problem: AI as a Logistics Game-Changer
While AI is revolutionizing combat systems, one of its most critical applications lies in solving military logistics challenges. A longstanding military adage states: “Romantics study tactics, but pragmatists study logistics.” This is no joke—logistics emerged as a formal discipline during World War II, when U.S. admirals faced the herculean task of supplying forces across the vast Pacific theater. With thousands of kilometers between resupply points and atolls serving as the only logistical nodes, they had to account for everything from ammunition to fresh water. Their success birthed modern logistics science.
Today’s battlefields present different challenges. The Monge-Kantorovich transport problem (optimal resource distribution under constraints) has become an ideal challenge for military AI. Unlike civilian logistics—where road networks remain stable and only traffic flow varies—war zones are dynamically destructive: bridges may be destroyed, supply routes cut by enemy forces, warehouses obliterated by missiles.
AI systems must continuously recalculate routes in real-time, minimizing exposure to threats while identifying alternative storage sites and delivery paths. This goes beyond simple navigation—it requires predictive threat analysis, resource allocation optimization, and adaptive contingency planning.
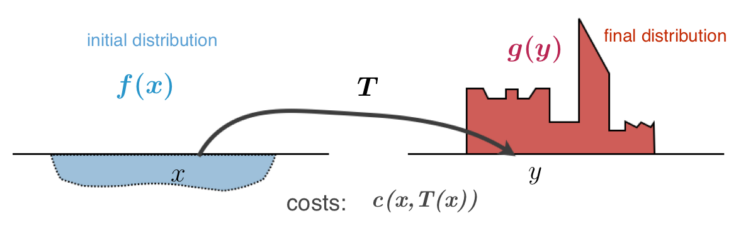
This dynamic necessitates highly complex calculations and the identification of optimal routes under conditions of near-total operational transparency: the adversary observes the concentration and movement of troop formations, the delivery of construction materials and ammunition to the front-line zone, and the maneuvering of reserves. Such transparency is achieved primarily through persistent reconnaissance conducted by drones and satellite surveillance. AI models may potentially optimize maneuvers to such an extent that, even when detected, the enemy lacks sufficient time to prepare.
The development of such applications is indirectly evidenced by events like the First International Forum on Digital Technologies in Transport and Logistics “Digital Transportation 2024”, held in October 2024, where AI-driven logistics optimization was a key topic of discussion.
Cryptography and AI: Who Will Crack Whom?
The advent of AI models has transformed cryptography. AI is no longer just a tool for encrypting and decrypting messages—it is now also used to “crack the user” (e.g., through deepfakes or phishing schemes to extract passwords or provoke destructive actions) and to compromise infrastructure, including the very AI models an organization relies on.
Yet, AI has also made significant strides in solving classical cryptographic challenges. By leveraging vast datasets, AI models can decipher various encryption methods through frequency analysis and keyword pattern recognition. These systems continuously learn and refine their capabilities. For instance, if a data breach exposes both encrypted and plaintext messages, passwords, or keywords, AI can use this information to train itself. Moreover, AI aggregates user activity data—such as public social media posts—to analyze writing patterns and later decrypt those same users’ encoded communications.
The competition between AI-driven offensive and defensive tools is a relentless arms race. Unlike large language models (such as ChatGPT), AI-assisted cryptography does not require the same massive computational power. Cryptographers can work with relatively standard supercomputers—and Russia has those in sufficient supply. That said, greater computing power always provides an edge.
Shortly after T-invariant published its investigation into MSU’s new “MGU-270” supercomputer, a high-performance computing (HPC) specialist familiar with the operations of MSU’s Research Computing Center (which oversees both the “Lomonosov-2” and “MGU-270” systems) reached out to the editorial team. According to T-invariant’s source, while the new machine was being assembled for MSU’s Institute of Artificial Intelligence—headed by Katerina Tikhonova—plans were also underway to develop a separate supercomputer for the FSB’s Institute of Cryptography, Communications, and Informatics (IKSI). However, the project was halted due to multiple complications. “Preparations began before the war—funding had been allocated for at least two new supercomputers, including one exclusively for IKSI,” the HPC expert explained. “But then everything fell apart: sanctions drove up component prices, procurement became harder, supply chains stretched, and in the end, there was only enough funding for one machine. To my knowledge, the idea of a dedicated cryptography supercomputer has been postponed for now.”
A representative from a Russian supercomputing firm suggested that IKSI specialists might now be running their computations on the MGU-270—a possible explanation for both the secrecy surrounding its development and the unprecedented restrictions on access. Unlike its predecessors (“Lomonosov” and “Lomonosov-2”), the MGU-270 is the first MSU supercomputer not openly available to researchers from other universities and institutes. (T-invariant previously detailed these computational restrictions, which mark a departure from MSU’s traditional policy of shared access.)
Background
The Institute of Cryptography, Communications, and Informatics (IKSI) traces its origins to the Higher School of Cryptographers, established on October 19, 1949, by a decree of the Politburo of the Central Committee of the Communist Party (VKP(b)). That same year, a classified department was created within Moscow State University’s Faculty of Mechanics and Mathematics to train specialists in the field.
These institutions were later reorganized into the Technical Faculty of the Felix Dzerzhinsky Higher School of the KGB (the USSR’s primary training academy for state security personnel). Following the dissolution of the Soviet Union, the faculty was restructured in 1992 into the Institute of Cryptography, Communications, and Informatics under the Academy of the FSB of Russia.
(Source: Official website of the FSB Academy)
“The FSB’s cryptographers are the intellectual elite. To an outsider, they’re indistinguishable from mathematicians at Moscow State University or researchers at any other institute,” says a T-invariant source familiar with the inner workings of IKSI. “Back in Soviet times, they were locked in a relentless rivalry with American specialists—a constant game of who could out-crack whom. Today, the competition is even fiercer, with entirely new technologies in play. It’s no surprise they wanted their own supercomputer. The military has its own resources—like the ‘Era’ technopolis or facilities ‘on the embankment’—but cryptographers need dedicated power too.”
“Sovereign AI”: What Does It Really Mean
Vladimir Putin’s calls for “sovereign artificial intelligence,” Katerina Tikhonova’s clarifications on its likely scope, the proliferation of closed-access supercomputers, and Denis Belousov’s restructuring of military drone production all point to one trajectory: Russia’s “sovereign AI” is, fundamentally, military AI.
Little is known about specific developments, but even observable clues—procurement patterns, institutional shifts, and hardware bottlenecks—suggest a clear priority: deploying AI models in warfare. Russia possesses both the computational resources (despite sanctions) and the technical expertise to pursue this. The only constraint? A shortage of advanced chips, critical not just for exaflop-scale computing but also for next-gen drones and robotics.
Yet workarounds exist, as seen with the MGU-270 supercomputer and gray-market import channels. This reality should give pause to both chip manufacturers and sanctions enforcers: barriers are being circumvented, and the technological gap may narrow faster than anticipated.